AI-driven technology can reduce duplicate patient records, overlays, and other patient identification issues
Patient safety depends on many factors. One of the most overlooked is the quality of data used to make clinical decisions. That data largely depends on how accurately patients are identified. Poorly identified patients are a primary cause of safety issues.
Two problems arise when patients are poorly identified:
- One results in patient duplication—multiple versions of the same patient with each version containing only a partial record. When a doctor treats a patient using a duplicated chart, they do not have all the information to make good clinical decisions.
- The second more dangerous problem occurs when one patient is confused with another, and a document is placed in an incorrect chart. This can lead clinicians to make erroneous diagnoses, prescribe incorrect medications, and perform the wrong surgeries.
What causes duplicate patient records?
During specimen accessioning, clinical laboratory staff rapidly enter patient information into a computer system. They have the difficult choice of quickly finding a patient previously tested or creating a new patient. Most accessioning systems lack quality search functions, so staff are likely to select the less risky option of creating a new patient. This results in significant duplication rates.
Even worse is when duplications and other patient identity errors spread to disparate environments. This can happen if a patient sees two or three doctors, each of whom uses a different clinical laboratory. Consequently, EHR records may not include every test result.
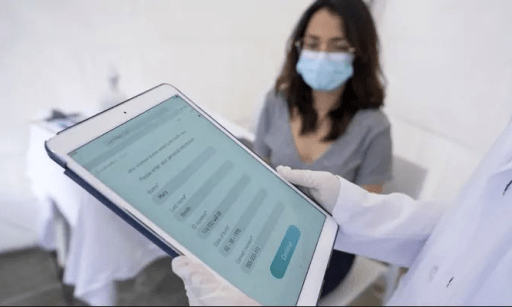
How do patient identification issues affect patient care?
Duplicate records can result in a doctor making decisions based on information from one lab without knowledge of tests from others. In 2012, researchers the University of Texas School of Biomedical Informatics conducted a retrospective, matched, cohort study of 904 events of abnormal laboratory results and concluded that “duplicate records are associated with a higher risk of missing important laboratory results when compared with non-duplicated records.”
More dangerous than chart duplications are “overlays,” which occur when one patient’s records end up in another’s chart. A clinician working with an overlay isn’t simply reading an incomplete record—they are reading entirely wrong medical information.
Given that 70 percent of clinical decisions are based on laboratory results, high-quality laboratory data should be a priority. Yet duplicate patient records, overlays, and other patient identification issues in clinical labs are among the worst problems in health care, largely possible due to an extraordinary amount of manual data entry. The inevitable outcome is low data quality.
AI-driven technology can improve the quality of patient data
The good news is that technology can help clinical labs improve data quality. Advances in AI and machine learning allow automation technology to reduce human intervention and error in patient matching.
In this type of workflow, a multilayered process first runs data through a master patient index, or MPI, to identify and merge obvious duplicates, while a second layer uses machine learning to correct errors. Referential matching and data enrichment then further reduce duplication. Lastly, data analytics resolves remaining duplicates and checks for overlays. Previous steps are then rerun. From there, clinical lab staff can address any unresolved questions.
Using intelligent automation to improve health data produces better results faster than manually performing the tasks. It also makes it more likely that clinicians have the information to provide the safest care.
This article was originally published by Today’s Clinical Lab and the original article can be viewed here.
Stay In Touch:
![]() | Thank you for Signing Up |


Stay In Touch:
![]() | Thank you for Signing Up |

