In the digital age, data plays a crucial role in shaping the healthcare landscape. Healthcare organizations generate vast amounts of data, ranging from electronic health records (EHR) to medical research and analytics. However, the quality of this data is paramount to the success and safety of patient care.
In this blog post, we will explore in-depth the significance of data quality in healthcare, the major problems caused by poor data quality, and the characteristics of a robust data quality management system.
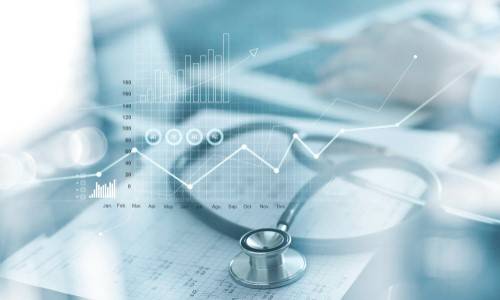
What is Data Quality Management?
Data Quality Management in healthcare is a systematic and ongoing process that ensures the accuracy, completeness, consistency, and reliability of data across various systems and applications. It involves a series of steps aimed at maintaining data integrity, including data collection, validation, integration, transformation, and cleansing. By implementing data quality management practices, healthcare organizations can trust the information they possess and make informed decisions based on reliable data.
Data quality management begins at the point of data entry, emphasizing the importance of accurate and complete information right from the start. This involves training and educating personnel to input data correctly, using standardized formats and predefined data entry rules. Data profiling tools are utilized to analyze and assess the quality of existing data, identifying any anomalies or inconsistencies that need to be addressed.
Moreover, data quality management is not a one-time activity; it requires ongoing maintenance and monitoring to ensure data remains accurate and up-to-date. Data stewards are appointed to oversee data quality initiatives and resolve issues as they arise. Regular data audits and data cleansing processes are performed to rectify errors, eliminate duplicates, and remove outdated information.
Incorporating data quality management as an integral part of the healthcare organization’s data governance strategy ensures that high-quality data is available for decision-making, research, and patient care.
The Value of Data Quality Management in Healthcare
Reliable Electronic Healthcare Records (EHR)
Electronic Health Records (EHRs) have revolutionized patient care by providing healthcare professionals with easy access to patient information. Ensuring the accuracy and completeness of EHRs through data quality management is essential for delivering optimal care. Accurate EHRs enable healthcare providers to have a comprehensive view of a patient’s medical history, medication details, lab results, and allergies, leading to more informed and personalized treatment decisions.
Additionally, data quality management ensures that EHRs are consistent across various systems and healthcare facilities, promoting seamless sharing of information among authorized parties, such as specialists and primary care physicians. This interoperability fosters collaboration and improves patient outcomes through coordinated care.
Timely Correct Diagnosis
Inaccurate or incomplete data can lead to delayed or incorrect diagnoses, impacting patient health and safety. By maintaining data accuracy and reliability, data quality management supports timely and precise diagnoses. Physicians can access up-to-date patient information, make accurate assessments, and initiate appropriate treatment plans promptly.
Furthermore, data quality management can aid in avoiding unnecessary diagnostic tests or treatments, reducing healthcare costs, and optimizing resource utilization.
Accurate Medical Research & Analytics
Data-driven medical research and analytics heavily depend on the accuracy and reliability of the underlying data. High-quality data serves as the foundation for meaningful insights, identifying patterns, and drawing conclusions. Researchers rely on clean and consistent data to draw valid conclusions, ensuring that medical discoveries and treatment protocols are evidence-based and effective.
Through data quality management, healthcare organizations can enhance the integrity of their research data, leading to advancements in medical knowledge and improved healthcare practices.
Patient Confidentiality
Patient confidentiality is a critical aspect of healthcare data management. Healthcare organizations are legally and ethically obligated to protect sensitive patient information from unauthorized access or disclosure. Data quality management plays a vital role in ensuring data security and privacy compliance, especially in the context of regulations like HIPAA and GDPR.
Robust data quality management practices include implementing stringent access controls, encryption, and data masking techniques to safeguard patient data from potential breaches and unauthorized use. By prioritizing data security, healthcare organizations can build and maintain trust with their patients, contributing to improved patient engagement and satisfaction.
Reliable HL7 Messaging for System Interoperability
Interoperability between different healthcare systems is crucial for seamless data exchange and efficient coordination of care. The Health Level 7 (HL7) messaging standards facilitate the secure and standardized exchange of clinical and administrative data between disparate healthcare applications.
Data quality management ensures that HL7 messages are accurate, complete, and adhere to the specified standards. When healthcare systems exchange reliable data through HL7 messaging, it reduces the risk of data inconsistencies or misinterpretations, resulting in improved patient safety and streamlined workflows.
Trustworthy Relationships Between Service Providers & Users
Patient confidentiality is a critical aspect of healthcare data management. Healthcare organizations are legally and ethically obligated to protect sensitive patient information from unauthorized access or disclosure. Data quality management plays a vital role in ensuring data security and privacy compliance, especially in the context of regulations like HIPAA and GDPR.
Robust data quality management practices include implementing stringent access controls, encryption, and data masking techniques to safeguard patient data from potential breaches and unauthorized use. By prioritizing data security, healthcare organizations can build and maintain trust with their patients, contributing to improved patient engagement and satisfaction.
Compliance
Healthcare organizations are subject to numerous data privacy and security regulations, such as HIPAA in the United States or GDPR in the European Union. Failure to comply with these regulations can result in severe penalties, legal consequences, and damage to the organization’s reputation.
Data quality management ensures that patient data is handled in accordance with these regulations, safeguarding patient rights and privacy. Implementing data quality management practices helps organizations meet compliance requirements, protecting both patients and the organization from potential data breaches or non-compliance issues.
Efficient Policy & Procedure Making
Data-driven policies and procedures are more effective in improving patient care and operational efficiency. Data quality management supports evidence-based decision-making by providing accurate and reliable data for analysis. Healthcare organizations can analyze performance metrics, patient outcomes, and resource utilization patterns to identify areas of improvement and implement evidence-based practices.
By continually monitoring data quality and analyzing key performance indicators, healthcare organizations can measure the impact of new policies and initiatives. This iterative approach to policy-making ensures that decisions are based on reliable data, leading to continuous improvements in patient care and operational efficiency.
4 Major Problems Caused by Poor Data Quality
Patient Frustration & Mistreatment
Inaccurate or incomplete data in healthcare can have serious consequences for patients. One of the most significant problems caused by poor data quality is misdiagnosis and inappropriate treatment decisions. When healthcare providers have access to inaccurate patient information, it can lead to incorrect diagnoses and treatment plans. Patients may undergo unnecessary procedures or receive treatments that are ineffective, causing frustration and distress. Moreover, delays in receiving the correct diagnosis and treatment can lead to prolonged suffering, exacerbate health conditions, and reduce patient confidence in the healthcare system.
For example, a patient with allergies or adverse reactions to specific medications may be prescribed a drug they are sensitive to, leading to adverse effects and prolonged recovery. Similarly, incorrect lab results or medical history may lead to a delayed diagnosis, giving the illness more time to progress before appropriate treatment is initiated.
To address this issue, healthcare organizations must prioritize data quality management to ensure that patient records are accurate, up-to-date, and comprehensive. Regular data validation and audits can help identify and rectify errors, ensuring that healthcare providers have access to reliable patient information for making informed decisions.
Employee Distrust of Critical Technology
Healthcare professionals heavily rely on technology to provide efficient and quality patient care. Clinical decision support systems, electronic health record (EHR) systems, medication administration tools, and other healthcare technologies play crucial roles in streamlining workflows and enhancing patient safety. However, when these technologies are based on poor data quality, healthcare professionals may lose trust in the system’s accuracy and reliability.
Distrust in critical technology can lead to resistance in adopting new digital tools or fully leveraging existing ones, hindering the potential benefits of healthcare technology advancements. When healthcare providers are unsure about the accuracy of the data provided by these systems, they may revert to manual processes, increasing the risk of errors and compromising patient safety.
To overcome this challenge, healthcare organizations must invest in data quality management strategies that ensure the integrity of data used in various technology applications. By establishing data governance frameworks, conducting regular data quality assessments, and involving healthcare professionals in the data validation process, organizations can build confidence in technology and encourage its effective use for improved patient care.
Decrease in Efficiency & Increase in Bottlenecks
Poor data quality can significantly impact the efficiency of healthcare processes, leading to delays and bottlenecks in patient care and administrative tasks. When healthcare providers and administrators work with inaccurate or inconsistent data, it can result in repetitive data entry and redundant tests. This inefficiency wastes valuable time and resources, diverting attention from patient care to administrative tasks.
For instance, when patient records contain duplicate entries or conflicting information, it requires additional effort to reconcile the data, delaying treatment decisions and causing frustration for both healthcare providers and patients. Additionally, errors in scheduling and resource allocation can lead to overbooked appointments or insufficient staffing, further exacerbating delays and inefficiencies.
To enhance efficiency, healthcare organizations should implement data quality management practices that focus on data validation and cleansing. By identifying and resolving data discrepancies and duplicates, healthcare providers can streamline workflows, optimize resource utilization, and improve overall operational efficiency.
Poor & Ineffective Policy Decisions
Data-driven policy decisions are fundamental to effective healthcare management. However, when decision-makers rely on inaccurate or incomplete data, it can lead to suboptimal patient care, resource misallocation, and ineffective policy-making.
For instance, if healthcare organizations use unreliable data to assess patient outcomes or resource utilization, they may overlook critical areas for improvement or invest resources in initiatives that yield limited benefits. Additionally, policy decisions based on faulty data may lead to inappropriate allocation of funds and services, resulting in higher healthcare costs and reduced quality of care.
To make effective policy decisions, healthcare organizations must establish a strong data quality management framework that encompasses data validation, profiling, and ongoing maintenance. By ensuring data accuracy and completeness, decision-makers can rely on high-quality data for informed policy-making, leading to improved patient care, optimized resource allocation, and more efficient healthcare operations.
Characteristics of Good Data Quality Management
Ensures Structure at the Source
Data quality management begins with capturing accurate and consistent data at the point of entry. To achieve this, a well-designed data quality management system establishes clear data entry standards and guidelines for data capture. This includes defining data formats, required fields, and permissible values to maintain uniformity and data integrity.
Implementing validation rules and automated data checks is essential to identify errors or inconsistencies during data entry. Real-time validation ensures that data meets predefined criteria and minimizes the chances of incorrect or incomplete information entering the system. Regularly updating validation rules based on evolving requirements and industry standards helps prevent data quality issues and ensures ongoing data accuracy.
By focusing on structure at the source, organizations can build a solid foundation for their data, streamlining downstream processes, and reducing the need for costly data cleansing efforts in the future.
Validates Data Formatting & Preconditions
Data validation is a critical step in data quality management, ensuring that data conforms to predefined criteria and business rules. It involves verifying data formatting, such as dates, numbers, and alphanumeric characters, to ensure consistency and standardization. Validation also includes assessing preconditions, ensuring that data meets specific prerequisites before being considered valid and usable.
For instance, in a patient registration form, validating that the date of birth is in the correct format and within reasonable age limits can help detect potential data entry errors. Similarly, in a laboratory test result, validating that the numerical values fall within expected ranges ensures the accuracy and reliability of the data.
Regularly updating validation rules based on changes in data requirements or regulatory standards is crucial to maintain data accuracy over time. Data profiling tools can assist in identifying data anomalies and inconsistencies, guiding data stewards in refining validation criteria to improve data quality.
Allows for Secure Collaboration
Data quality management extends beyond data capture and validation; it also involves secure data sharing and collaboration among authorized stakeholders. A robust data quality management system ensures that data is accessible to the right people while maintaining strict controls to prevent unauthorized access or data breaches.
Role-based access controls determine who can access, view, and modify specific data elements based on their job roles and responsibilities. Additionally, encryption mechanisms can protect sensitive data during transmission, ensuring its confidentiality.
Interoperability with other systems and applications is essential for seamless data exchange within healthcare organizations and across healthcare networks. Implementing secure and standardized data exchange protocols enables reliable data sharing while safeguarding patient privacy and data integrity.
By fostering secure collaboration, data quality management supports effective communication and decision-making, leading to improved patient care and outcomes.
Has Tools for Ongoing Maintenance and Profiling
Data quality management is not a one-time effort but an ongoing process. Regular data profiling and maintenance are crucial to identify and address data quality issues proactively.
Data profiling tools analyze data sets to reveal patterns, inconsistencies, and outliers, providing insights into data quality levels. Data stewards can use this information to identify potential data quality issues and take corrective actions promptly. By understanding data quality trends, organizations can identify areas that require improvement and prioritize data cleansing and enrichment efforts.
Automated data maintenance processes, such as data cleansing and data enrichment, play a significant role in keeping data accurate and up-to-date. Data cleansing involves identifying and correcting errors, duplicates, or outdated information, while data enrichment involves enhancing data with additional relevant information from reliable sources.
Continuous data maintenance and profiling are essential for maintaining data accuracy and ensuring that data remains fit for use in critical decision-making processes.
Properly Accounts for Data Lifecycle
Data quality management should encompass the entire data lifecycle, from data creation to archival or disposal. Data quality requirements may vary based on the stage of the data lifecycle and its intended use.
Data creation involves capturing accurate and complete data at the point of entry, as discussed earlier. As data evolves and becomes part of various processes, data quality management must ensure data integrity and consistency across all stages.
Data retention policies and procedures are essential to govern data storage and preservation. Organizations must define data retention periods based on legal, regulatory, and business requirements. Ensuring compliance with data regulations, such as HIPAA or GDPR, is crucial during data storage and retention.
Proper data disposal and archiving are equally important to prevent the accumulation of obsolete or irrelevant data. Organizations should have protocols for securely archiving historical data and safely disposing of data that is no longer required. Archiving historical data maintains data integrity while reducing data clutter, making it easier to access and manage relevant information.
By properly accounting for the data lifecycle, organizations can maintain data accuracy and relevance, reduce storage costs, and ensure compliance with data regulations throughout the data’s lifecycle.
Premier Data Quality Management Solutions
4medica’s data quality management solutions are specifically designed to meet the unique challenges faced by healthcare organizations. We ensure data is captured accurately from the start with data entry standards and validation rules. Our advanced data validation checks ensure data formatting and preconditions adhere to industry standards. Security is paramount, with role-based access controls and encryption mechanisms for secure collaboration.
We understand that data quality is an ongoing process, and our data profiling tools provide valuable insights to address issues proactively. Automated data maintenance, including cleansing and enrichment, ensures data accuracy and relevance.
With 4medica’s data quality services, healthcare organizations can rely on trustworthy data for critical decision-making, optimize patient care, and improve overall efficiency, ultimately providing exceptional healthcare experiences to patients.